Life Cycle Assessment is not Rocket Science.
The author Dan Goleman once quoted me as having told him “Life Cycle Assessment is not rocket science.” And I joked that I felt able to say this in part because I had earlier worked at the Rocket Propulsion Lab in the California Desert. As I explained to Dan then, and as I share with a new crop of students every Fall semester, rather than being “rocket science”, LCA is primarily “a data problem” in two related ways.
The first is that for many – indeed still for most – processes in the economy, we lack LCA data to explicitly model them. This is especially true if you need to be specific about the geography and the technology, and if you want up-to-data data. Despite the fact that the world has been doing LCAs since the late 1960s, there are huge data gaps in humanity’s LCA data resource base.

The second data problem with LCA is, surprisingly, the reverse of the first problem! There are areas of the economy where we have, you could say, “too much data” or too many options. What makes this situation challenging rather than simply a case of being well-provisioned is that users lack a reliable or informative basis for selecting the most appropriate data, the best available data to model what it is they really want to model.
I have started to call these two extremes “data deserts” and “data swamps.” In a data desert, you find yourself alarmingly far from any essential resource, and you wonder how you can go on. In a data swamp, you are tangled up to your eyeballs in a confusing and paralyzing morass of data options, not able to make proper headway. Neither situation, neither experience, is the least bit pleasant, I can tell you. A significant portion of learning the “art” of LCA these days is learning how to navigate through these two challenging situations that are too often the norm.
The problem above gives some cover to those LCA consultants who have a tendency to tell their clients:
Don’t try this at home. Leave LCA to the white-smocked professionals.
But here’s the thing that bounces me out of bed these days: a combination of intelligent software, data science, and good old fashioned human teamwork is about to convert this inhospitable terrain into verdant data forests and plains, places where many, most, and eventually all of us can freely roam, find the answers we need, and leave the land even more beautiful than we found it. While we might still want to invite a consultant guide to help us tackle some serious modeling adventures from time to time, most of us will most of the time be able to capably explore the data terrain on our own, and in ways that help one another.
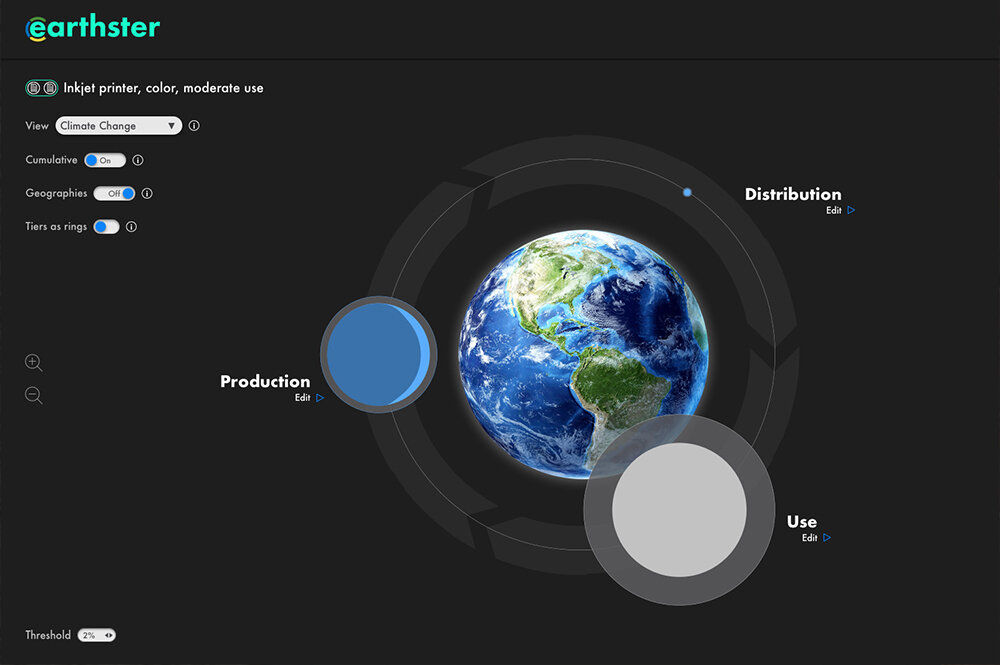
I’ll describe the software and data science part of the solution first, and the teamwork part last.
Earthster software is using data science to tell users, no matter where they are on the data map: here is the best available data for your intended application. In this case, “best available” simply means “lowest possible uncertain in practice.” In this way, the software will show you that you are never in an absolutely barren data desert. There is always some data to be made use of, for your intended application, and the task is to quantify the uncertainty that arises because of the data imperfections. In many cases, while the results may be uncertain, you can still draw some very useful conclusions from your analysis. And in all cases, you can identify the most influential uncertainties active in your specific application, clarifying the highest priorities for follow-up data collection.
Software and data science also help you to move quickly and nimbly through data swamps. And again, uncertainty is surprisingly your friend. In the swamp, we simply ask: which of the bewilderingly many data options will provide me with lowest-uncertainty results. From this basic question, one data option emerges as the preferred solution, and your model comes into focus.
Armed with explicit treatment of uncertainty, you no longer have to fear data deserts nor data swamps. You can roam the data terrain confidently, and we’ll all be better off when we all can and do. In a system such as Earthster, every step you take in the data terrain leaves a positive trace. As you come to understand your own processes better, you are building yourself a data-driven model of your particular location in data space.
The resulting insights can be shared in ways that protect your confidential information; one way is by averaging your results with those of similar economic activities. The average can be shared with the world, clarifying your zone of the map without revealing your confidential coordinates if you don’t want to.
This average is immediately useful to you as a basis for benchmarking. And it is helpful to the world as it fills in one more region that used to be only sparsely understood, converting one more data-arid zone to a more fruitful and fertile data-scape.
Go ahead, try this at home. You’ll find it safe and productive. And the more the merrier.